The country is struggling with extended NHS wait times for treatments, but new artificial intelligence and analytic solutions could be used to help resolve the crisis
In 2022, the number of patients on massive lists with long NHS wait times rose to a record seven million after continued nursing strikes and a combined flu and COVID winter.
A significant factor contributing to bottlenecks is a lack of hospital beds, however, there’s an array of factors compounding the issue. The bottom line is NHS wait times and delays to treatment risk harm to those in need of attention, and create further backlogs in hospitals.
The situation has continued to worsen with the government announcing that the NHS will block book thousands of beds in residential care homes. A step that comes at a massive cost both directly in fees and also logistically.
To deliver meaningful change, the government must consider leveraging new technologies. Last month we saw the NHS rolling out pilot schemes to use bots to prioritise those waiting for operations. However, an end-to-end approach that capitalises on artificial intelligence (AI) and cloud analytics is needed.
The government must consider leveraging new technologies
Bringing together data within integrated care systems for the best results
To use AI and analytics effectively to decrease NHS wait times, it’s important that the whole patient journey can be mapped out. To do so we must harness the data across all branches of the health and social care system and create a holistic view of the situation, beyond just treatment lists.
In July 2022, 42 integrated care systems (ICS) were introduced in the UK, aiming to connect local health and care branches into a single organisation. They have been designed to improve local healthcare, tackle inequality, enhance productivity and help the NHS to support broader social and economic development by bringing multidisciplinary teams together.
Before integration, each organisation had worked in silos, using their own software, each with different data storage programs and workflows. Although the care systems are now technically integrated, in many instances large datasets and complicated processes remain incompatible.
As more extensive data is available, such as patient history from both medical and social records, the benefits of analytic modelling increase. The data is more comprehensive and the outcomes reflect this by providing more bespoke insights. AI is key to overcoming the issues associated with disjointed data, ultimately to derive the most value from cloud analytics solutions.
Machine learning programs can be developed to seek out, translate, and file important information from across the health, social, and mental health services into one. This would bring together not only health data but also socioeconomic and political factors, which would prove beneficial for the more vulnerable and deprived in society.
How AI can reduce the issue of bed blocking
Bed blocking is a major factor contributing to wait times in NHS hospitals. In fact, one NHS Confederation study revealed that on an average day in September 2022, of the 22,300 patients that were medically eligible for a discharge more than 13,000 remained in the hospital due to a lack of available social care packages.
With the right tools it is possible to reduce or even prevent bottlenecking due to a lack of social care packages. Using advanced analytics, the expected time before a patient is fit for discharge and arranging their likely social care needs – including the provision of wheelchairs and required care in the community – can be predicted. AI can then suggest more efficient allocation and scheduling of resources, to ensure care needs can be met when the patient is fit for discharge.
Not only will this reduce the administration needs of social care workers but it will provide them with deeper, more expansive insights. Health and social care workers are then able to focus on delivering the necessary care and support, instead of spending time organising packages.
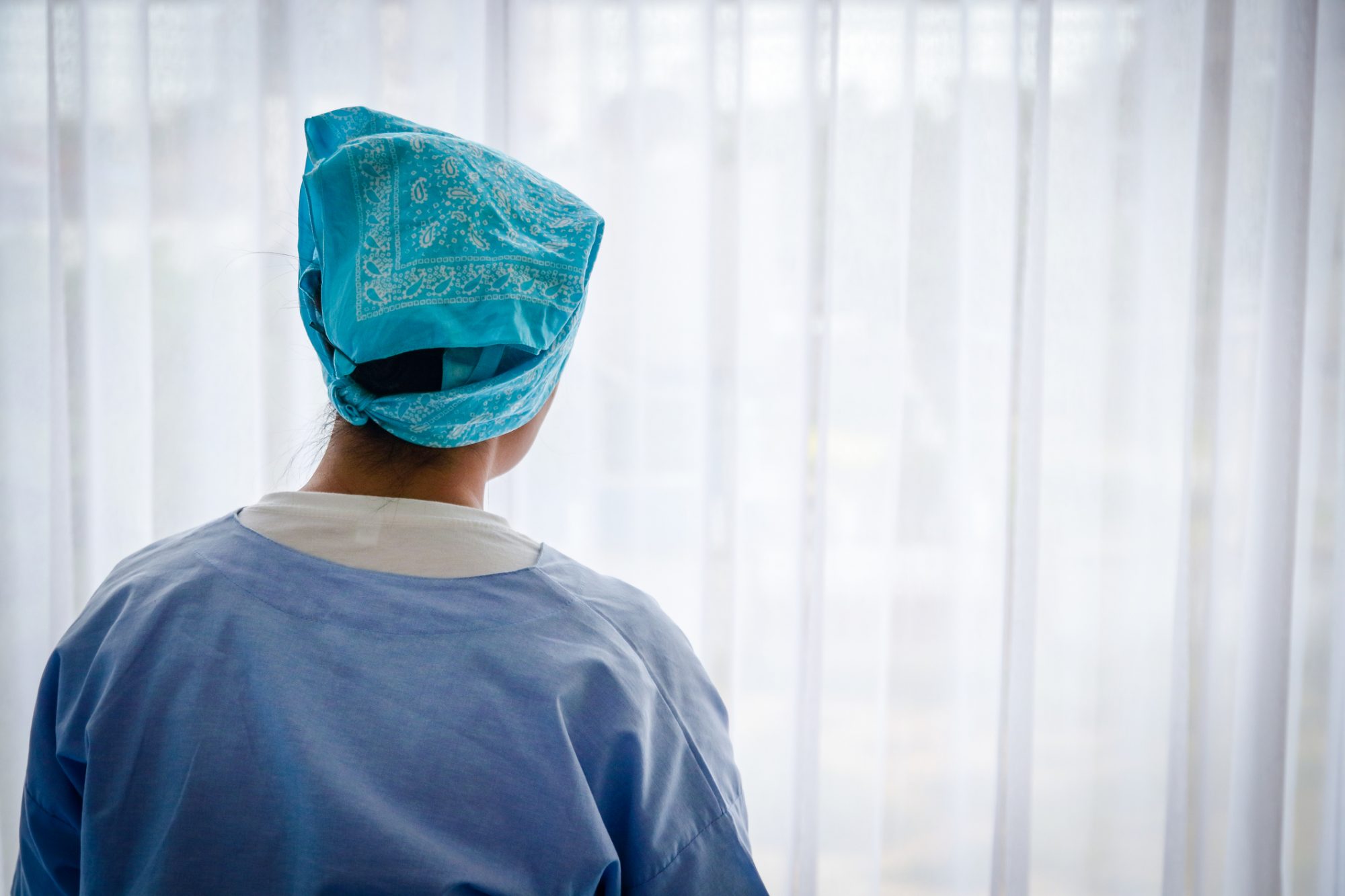
Creating a system of trust
Trust is a vital factor. Already there are concerns about patient reaction to the NHS’ rollout of surgery prioritisation bots, fearing that the automated calls will be distrusted. As the systems become more complex and cover a wider range of functions, there are some concerns about public confidence.
Championing clarity for the millions who will interact with the system, from the first instance, should therefore be just as significant a focus as their design.
For healthcare workers the same applies. To safeguard patients and gain the trust of those delivering healthcare, the decisions made by AI must be transparent and able to stand up to scrutiny before they are applied. This relies on the route to outcomes being explainable and based on clear data.
Analytics platforms are only as good as the data they have available, so “boots on the ground” will be needed to interact with the program. For this reason, any solution must be thoroughly thought out. If the system isn’t trusted or is unintuitive it will increase the pressure on busy teams and uptake of the system will suffer, leading to missed potential.
A tool for equality
Ensuring that AI and analytics platforms are ethical must be a cornerstone of development. A common misconception is that removing bias from AI systems is the path to equality. In fact, we must sometimes factor in reverse bias as a counterweight to the real-world disadvantages faced by certain groups.
Those from ethnic minority groups face longer wait times for treatment
NHS watchdog HealthWatch has pointed out that those from ethnic minority groups face longer wait times for treatment. It is imperative that systems put in place do not emphasise this bias. Instead, we must use new tools to work preventatively.
Providing solutions for the future
The integration of advanced technologies into the NHS will create the opportunity for more jobs and upskilling of current workers. More than 100,000 NHS staff could now be trained in coding and bot creation if the bot systems, which are currently being trialled in areas including Lancashire, South Cumbria and Blackpool, are fully rolled out.
The factors contributing to the NHS wait list crisis are vast, and complicated, and cannot be fixed overnight. The consequences not only affect those waiting for treatment but also contribute to the strain on the service’s personnel and resources. Employing the right AI and analytics tools in a sensible, ethical, and unified way across the whole health and social care sector will provide a strong foundation to impact much-needed change.
By Mark Frankish, Data Scientist, SAS UK